GRANULAR MATERIALS FOR NEUROMORPHIC CIRCUIT APPLICATIONS
In this theme we are putting our knowledge and skills at the service of granular materials for applications in the field of neuromorphic circuits, for new computer architectures inspired by the brain. These architectures must now integrate processing and memory for faster, more efficient, computation, ideal for AI applications such as deep learning and pattern recognition.
At present, modern computers based on CMOS binary logic and von Neumann architecture will rapidly become ineffective in coping with the revolution caused by the growing amount of data. A transition to a new paradigm of brain-inspired computing systems based on adaptive nanoscale devices capable of emulating the brain’s biological processes is absolutely necessary. These systems must include a large-scale network of artificial neurons and plastic synapses, and enable spike-based communication and data processing through the manipulation of synaptic weight [1].
So the challenges of this neuromorphic computing approach are to find the key materials that include synaptors and neuristors. Memristors, non-volatile memories made from phase-change memories such as PCMs, mimic synaptic behaviour by changing state via nanoscale structural modifications or phase transitions. Neuristors, on the other hand, mimic neuron firing by using memristors and resistor-capacitor circuits that reproduce the LIF (Leaky, Integrate, and Fire) model.
We are therefore developing two complementary approaches:
1 – Study of intermediate states (IRS) in phase change memories (PCM) (collaboration with CEMES, STMicroélectronics and LETI):
This area is being developed as part of the ANR INTERSTATES (leader: CEMES) with our colleagues and partners at CEMES, LETI and STMicroélectronics. In this project, we are combining two research groups (NANOTECH LPCNO and MEM CEMES) with STMicroelectronics as industrial partner, with complementary expertise offering a unique opportunity to explore the IRS of Ge rich GST (G-GST) PCMs. The two multidisciplinary research teams (physicists, materials, electrical characterisation) from the LPCNO and CEMES laboratories share complementary skills and equipment.
Phase change memories (PCMs) are very good candidates for such building blocks [2-4]. Phase-change memories based on GST-225 exhibit accumulative behaviour, with a conductance that can be progressively increased or decreased by a train of electrical pulses (Figure). Their resistance values, intermediate between those of the SET (crystalline) and RESET (amorphous) configurations, could be used as synaptic weights [5-7] if they are stable over time, allowing PCMs to mimic synaptic activity and offering adjustable plasticity [6-7].
Although several authors have stipulated that the continuous changes in conductivity of PCM could be due to the increase/decrease of the fraction of crystalline nuclei in the amorphous matrix until the percolation paths progressively connect/disconnect the electrodes [8-10], no physical evidence has been identified so far.
This hypothesis is probably sufficient to explain the conductivity changes observed on a congruent material such as GST-225, but certainly not relevant for materials such as G-GST which decompose and form different phases during crystallisation, due to the high concentration of germanium in the amorphous material as deposited. Thanks to a multi-stage crystallisation scenario, G-GST and NG-GST materials are good candidates for use in memory devices to achieve brain-inspired computing applications with an optimised programming window and high thermal stability.
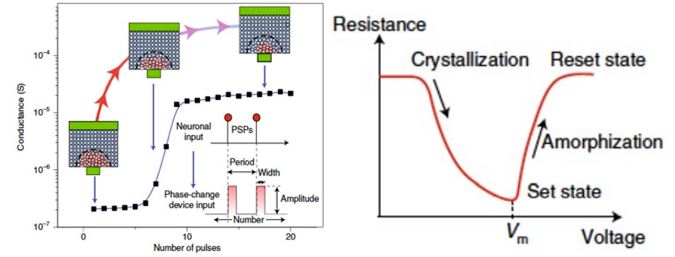
We want to demonstrate the possibility of programming G-GST-based PCM devices in different intermediate resistance states (IRS) with low resistance drift over time. Multilevel cells programmable by trains of electrical pulses are key elements of neuromorphic computing. Unlike revolutionary approaches that may lack production prospects, this project is evolutionary and aims to explore the possibility of propelling an advanced digital platform fabricated in high volume using state-of-the-art technologies (28nm FDSOI) into the bio-inspired era.
A thorough understanding of the physical properties (from the atomic to the device scale) of these SRIs is required to successfully control their formation. The spatial distribution of the Ge and GST phases must be known and controlled at each stage of progressive switching, in order to control the progressive opening of stable conduction paths and to assess the characteristics of the SRI.
This project should provide STMicroelectronics with a programming strategy that can be used to achieve the largest possible number of well-distinguishable SRIs that are stable over time and temperature.
The project has three interdependent objectives:
1- To understand how the structure of the G-GST layer of a PCM can be manipulated at the nanoscale to mesoscale, by adjusting the electrical stimuli applied to the PCM. Achieving this objective will allow us to electrically control the size, phase and relative organisation of the individual crystalline grains and remaining amorphous material when the G-GST layer is in a structural state intermediate between the SET and RESET states of the PCM.
2- To understand the origin, at the nanometric to mesoscopic scale, of the electrical properties of the intermediate states of G-GST PCM. In particular, to understand the close relationship between the structural/chemical characteristics (e.g. size, phase, interconnection of grains of different phases) and the electrical conduction mechanisms (conduction paths through the G-GST layer) of successive intermediate states of PCM devices.
3- Find the best programming strategies that can be used to switch G-GST PCMs into different intermediate states that have the desired electrical resistivity values and good stability over time and temperature.
2 – Towards neuromorphic circuits: (Collaboration with S. Tricard (LPCNO), S. Brown (School of Physical and Chemical Sciences, Canterbury University, New Zealand),
In this area, with my colleague Simon Tricard (NCO LPCNO team), we are developing a combined chemistry/physics approach to study the modulation of the electrical properties of hybrid metallic nanoparticle/functional molecule materials, which could be applied to the field of neuromorphics. Recently, we obtained funding from the PHC program Dumont d’Urville to initiate a collaboration with Dr Simon Brown from the School of Physical and Chemical Sciences, Canterbury University, New Zealand, one of the world’s leading specialists in neuromorphic materials. This contact opens up extremely promising prospects for the development of low-power electronic devices. The strength of this consortium lies in the synergy between our world-renowned teams, thanks to the complementary expertise in chemistry, physical measurements and modelling of the various partners, to define a resolutely new approach to the concepts of neuromorphic electronics. Today, the whole world depends on access to computer technology, even for the simplest everyday tasks. The internet, mobile phones and even fridges are built using modern computer chips whose power derives from incredible improvements in chip manufacturing technologies.
It was therefore surprising for many to discover that the IT industry is still facing two major crises:
1) there are entrenched bottlenecks that prevent further improvements in standard computer chips, and
2) IT consumes large amounts of energy (~10% of the world’s electricity) and generates huge amounts of CO2.
These figures are increasing dramatically year on year and are likely to be exacerbated by artificial intelligence (AI), which generally relies on large supercomputers.
The solutions to both these crises lie in one place: the human brain:
1) The structure of the brain bypasses the bottlenecks of existing computing technologies by using complex hierarchical architectures, rather than regular arrays of transistors, and
2) the brain is incredibly powerful at performing many computations, many computing tasks (e.g. pattern recognition), using remarkably little power (<50W).
That’s why researchers around the world are taking inspiration from the way the brain works to explore new brain-like (‘neuromorphic’) technologies. One of the most promising strategies is to take advantage of the intrinsically neuron- and synapse-like behaviour of certain classes of new nanoelectronic devices.
Our New Zealand partner, Dr Simon Brown, has developed a unique and powerful ability to construct nanoparticle arrays that exhibit brain-like behaviour, in particular by demonstrating that the spaces between nanoparticles act like neurons, and that, as in the brain, avalanches of neuronal spikes are critical, i.e. the arrays are optimised for information processing.
More recently, French partners Simon Tricard (NCO/LPCNO) and I have demonstrated that the insertion of well-designed molecules is a very powerful tool for regulating the electrical properties of nanoparticle assemblies [11] with properties similar to those of neurons and synapses. The devices in question are self-assembled using simple, low-cost methods and operate at speeds around 1000 times faster than the brain.
These techniques are therefore ideal for building brain-like networks, enabling existing artificial intelligence technologies to be reproduced on chips that can be incorporated into a multitude of much smaller and more efficient commercial electronic devices (e.g. wearable computers, medical implants, the Internet of Things, remote environmental monitoring, etc).
The aims of the project are as follows:
– The manufacture of electronic devices based on hybrid materials combining Dr Simon Brown’s nanoparticles and Dr Simon Tricard’s molecules,
– Electrical characterisation using impedance spectroscopy and demonstration of neuromorphic behaviour,
– Development of system modelling such as a percolation network.
The main objective of this project is therefore to build neuromorphic devices in which interesting molecular species are deposited on nanoparticle arrays:
1) to demonstrate new types of brain-like functionality and
2) to understand how new functionalities can be incorporated into devices with improved computational performance.
Specific computations targeted include associative learning via the formation of pathways through networks, and unsupervised learning tasks (where inputs to the network are basic data).
1 – S. Yu, Proceedings of the IEEE 106, 260 (2018).
2 – D. Ielmini et al., Nature Electronics 1, 333 (2018).
3 – R. Islam, J. Phys. D : Appl. Phys. 52, 113001 (2019).
4 – Memristive devices for brain-inspired computing, Woodhead Publish. Series in Electronics and Optical Materials, S. Spiga et al. editors, Elsevier (2021).
5 – G. W. Burr et al., Advances in Physics: X 2, 89 (2017).
6 – Phase change memory device physics, reliability and applications, Andrea Redaelli editor, Springer (2018).
7 – P. Zuliani et al, IEEE Transactions on Electron Devices 60, 4020 (2013).
8 – G. Navarro et al., 2013 IEEE IEDM meeting, p.21.5.1 (2013).
9 – M. A. Luong et al., Phys. Stat. Sol. RRL 2000443 (2020).
10 – Y. Yin, Appl. Phys. Lett. 95, 133503 (2009).
11 – Enhanced Dielectric Relaxation in Self-Organized Layers of Polypeptides Coupled to Platinum Nanoparticles: Temperature Dependence and Effect of Bias Voltage. Louis Merle, Ghada Manai, Adeline Pham, Sébastien Lecommandoux, Philippe Demont, Colin Bonduelle, Simon Tricard, Adnen Mlayah, Jérémie Grisolia* J. Phys. Chem. C 2021, 125, 41, 22643–22649 https://doi.org/10.1021/acs.jpcc.1c06457